Mynlp介绍
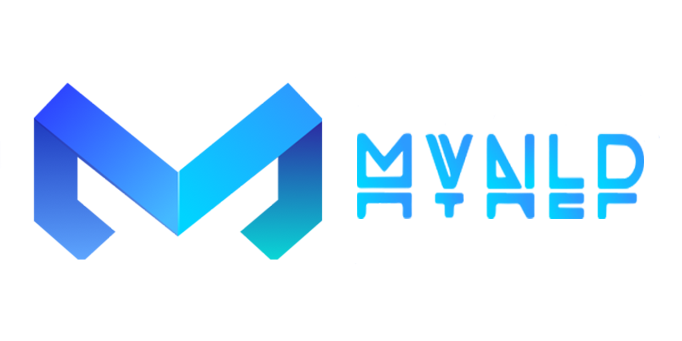
MYNLP是一个Java实现的高性能、柔性API、可扩展的中文NLP工具包。
-
感知机分词
-
CORE二元语言模型&词典分词
-
词性标注
-
通用感知机
-
命名实体识别(人名、地名、组织机构名)
-
fastText
-
文本分类
-
新词发现
-
拼音转换&切分
-
简繁体转换
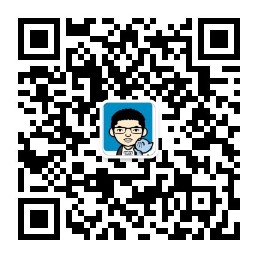
快速入门
该章节介绍如何安装和简单使用mynlp的基础功能。
安装
mynlp已经发布在Maven中央仓库中,所以只需要在Maven或者Gradle中引入mynlp.jar依赖即可。
compile 'com.mayabot.mynlp:mynlp:3.2.1'
<dependency>
<groupId>com.mayabot.mynlp</groupId>
<artifactId>mynlp</artifactId>
<version>3.2.1</version>
</dependency>
因为资源文件较大,所以mynlp.jar包默认不包括资源文件(词典和模型文件)依赖。
懒人方案,通过引用mynlp-all依赖默认提供的资源词典,满足大部分需求。
compile 'com.mayabot.mynlp:mynlp-all:3.2.1'
词典和模型资源
Gradle 坐标 | mynlp-all依赖 | 文件大小 | 说明 |
---|---|---|---|
com.mayabot.mynlp.resource:mynlp-resource-coredict:1.0.0 |
Y |
18.2M |
核心词典(20w+词,500w+二元) |
com.mayabot.mynlp.resource:mynlp-resource-pos:1.0.0 |
Y |
17.5M |
词性标注模型(感知机模型) |
com.mayabot.mynlp.resource:mynlp-resource-ner:1.0.0 |
Y |
13.4M |
命名实体识别(人名识别、其他NER) |
com.mayabot.mynlp.resource:mynlp-resource-pinyin:1.1.0 |
Y |
272K |
拼音词典、拼音切分模型 |
com.mayabot.mynlp.resource:mynlp-resource-transform:1.0.0 |
Y |
478K |
繁简体词典 |
com.mayabot.mynlp.resource:mynlp-resource-cws:1.0.0 |
N |
62.4M |
感知机分词模型 |
com.mayabot.mynlp.resource:mynlp-resource-custom:1.0.0 |
N |
2.19M |
自定义扩展词库 |
根据实际的需要,按需引入资源包。
compile 'com.mayabot.mynlp:mynlp:3.2.0'
// 核心词典
implementation 'com.mayabot.mynlp.resource:mynlp-resource-coredict:1.0.0'
// 词性标注
implementation 'com.mayabot.mynlp.resource:mynlp-resource-pos:1.0.0'
// 命名实体
implementation 'com.mayabot.mynlp.resource:mynlp-resource-ner:1.0.0'
// 拼音
implementation 'com.mayabot.mynlp.resource:mynlp-resource-pinyin:1.1.0'
// 繁简体转换
implementation 'com.mayabot.mynlp.resource:mynlp-resource-transform:1.0.0'
// 感知机分词模型
// implementation 'com.mayabot.mynlp.resource:mynlp-resource-cws:1.0.0'
// 自定义扩展词库
// implementation 'com.mayabot.mynlp.resource:mynlp-resource-custom:1.0.0'
基本用法
中文分词
Lexer是一个词法分析器的接口,通过Builder可以构建不同功能的分词器。
词法分析包括分词、词性标注、实体识别。
CORE分词器
CORE分词器是基于词典和二元语言模型的分词算法实现。
Lexer lexer = Lexers.coreBuilder() (1)
.withPos() (2)
.withPersonName() (3)
.build();
Sentence sentence = lexer.scan("mynlp是mayabot开源的中文NLP工具包。");
System.out.println(sentence.toList());
1 | CORE分词器构建器 |
2 | 开启词性标注功能 |
3 | 开启人名识别功能 |
[mynlp/x, 是/v, mayabot/x, 开源/v, 的/u, 中文/nz, nlp/x, 工具包/n, 。/w]
感知机分词
感知机分词器是基于BEMS标注的,结构化感知机分词算法实现。
Lexer lexer = Lexers
.perceptronBuilder()(1)
.withPos()
.withPersonName()
.withNer()(2)
.build();
System.out.println(lexer.scan("2001年,他还在纽约医学院工作时,在英国学术刊物《自然》上发表一篇论文"));
1 | 感知机分词器 |
2 | 开启命名实体识别 |
2001年/t ,/w 他/r 还/d 在/p 纽约医学院/nt 工作/n 时/t ,/w 在/p 英国/ns 学术/n 刊物/n 《/w 自然/d 》/w 上/f 发表/v 一/m 篇/q 论文/n
Pipeline插件示例
Lexer是基于Pipeline结构实现的,通过Plugin机制可以任意扩展Lexer的功能和行为。下面的实例演示了自定义词典的插件。
MemCustomDictionary dictionary = new MemCustomDictionary();(1)
dictionary.addWord("逛吃");
dictionary.rebuild(); (2)
FluentLexerBuilder builder = Lexers.coreBuilder()
.withPos()
.withPersonName();
builder.with(new CustomDictionaryPlugin(dictionary));(3)
Lexer lexer = builder.build();
System.out.println(lexer.scan("逛吃行动小组成立"));
1 | 一个自定义词典的实现 |
2 | 词典需要rebuild生效 |
3 | 配置CustomDictionaryPlugin插件 |
拼音转换
中文转拼音
PinyinResult result = Pinyins.convert("招商银行,推出朝朝盈理财产品");
System.out.println(result.asString());(1)
System.out.println(result.asHeadString(","));(2)
result.fuzzy(true);(3)
System.out.println(result.fuzzy(true).asString());
result.keepPunctuation(true);(4)
//result.keepAlpha(true);
//result.keepNum(true);
//result.keepOthers(true);
System.out.println(result.asString());
1 | 完整拼音字符串 |
2 | 只输出拼音首字母,逗号分隔 |
3 | 输出模糊拼音后鼻音等 |
4 | 保留标点 |
zhao shang yin hang tui chu chao chao ying li cai chan pin z,s,y,h,t,c,c,c,y,l,c,c,p zao sang yin han tui cu cao cao yin li cai can pin zao sang yin han , tui cu cao cao yin li cai can pin
拼音流切分
拼音流切分是指,将连续的拼音字母切分为一个一个原子单位。
System.out.println(PinyinSplits.split("nizhidaowozaishuoshenmema"));
[ni, zhi, dao, wo, zai, shuo, shen, me, ma]
文本分类
mynlp采用fasttext算法提供文本分类功能,你可以训练、评估自己的分类模型。
训练数据是个纯文本文件,每一行一条数据,词之间使用空格分开,每一行必须包含至少一个label标签。默认 情况下,是一个带`label`前缀的字符串。
__label__tag1 saints rally to beat 49ers the new orleans saints survived it all hurricane ivan __label__积极 这个 商品 很 好 用 。
所以你的训练语料需要提前进行分词预处理。
// 训练参数
InputArgs trainArgs = new InputArgs();
trainArgs.setLoss(LossName.hs);
trainArgs.setEpoch(10);
trainArgs.setDim(100);
trainArgs.setLr(0.2);
FastText fastText = FastText.trainSupervised(trainFile, trainArgs);(1)
FastText qFastText = fastText.quantize(); (2)
//fastText.saveModel("example.data/hotel.model");(3)
fastText.test(testFile,1,0.0f,true);(4)
System.out.println("--------------");
qFastText.test(testFile,1,0.0f,true);
1 | 训练一个分类模型 |
2 | 使用乘积量化压缩模型 |
3 | 保存模型文件 |
4 | 使用测试数据评估模型 |
Read file build dictionary ... Read 0M words Number of words: 14339 Number of labels: 2 Number of wordHash2Id: 19121 Progress: 100.00% words/sec/thread: Infinity arg.loss: 0.22259 Train use time 790 ms pq 100% compute_codes... compute_codes success F1-Score : 0.915167 Precision : 0.903553 Recall : 0.927083 __label__neg F1-Score : 0.919708 Precision : 0.931034 Recall : 0.908654 __label__pos N 400 P@1 0.918 R@1 0.918 -------------- F1-Score : 0.917526 Precision : 0.908163 Recall : 0.927083 __label__neg F1-Score : 0.922330 Precision : 0.931373 Recall : 0.913462 __label__pos N 400 P@1 0.920 R@1 0.920
简繁转换
Simplified2Traditional s2t = TransformService.simplified2Traditional();
System.out.println(s2t.transform("软件和体育的艺术"));
Traditional2Simplified t2s = TransformService.traditional2Simplified();
System.out.println(t2s.transform("軟件和體育的藝術"));
軟件和體育的藝術 软件和体育的艺术
简单文本摘要
文本摘要包含了两个简单TextRank的实现。
KeywordSummary keywordSummary = new KeywordSummary();
keywordSummary.keyword("text",10);
SentenceSummary sentenceSummary = new SentenceSummary();
List<String> result = sentenceSummary.summarySentences(document, 10);
KeywordSummary和SentenceSummary内置了默认的分词实现,你可以配置自定义的Lexer对象,参加具体文档。
中文分词、词性标注、命名实体
lexer架构
分词、词性、命名实体这三个任务一起被称为 词法分析
,mynlp中使用Lexer接口这个功能进行定义。
Lexer负责对有限短的文本(一句话、一个段落)进行词法分析。
nlp中有各种各样的分词算法,mynlp并没有为每个算法定义一个分词器类,而是使用Pipeline方式进行组装。
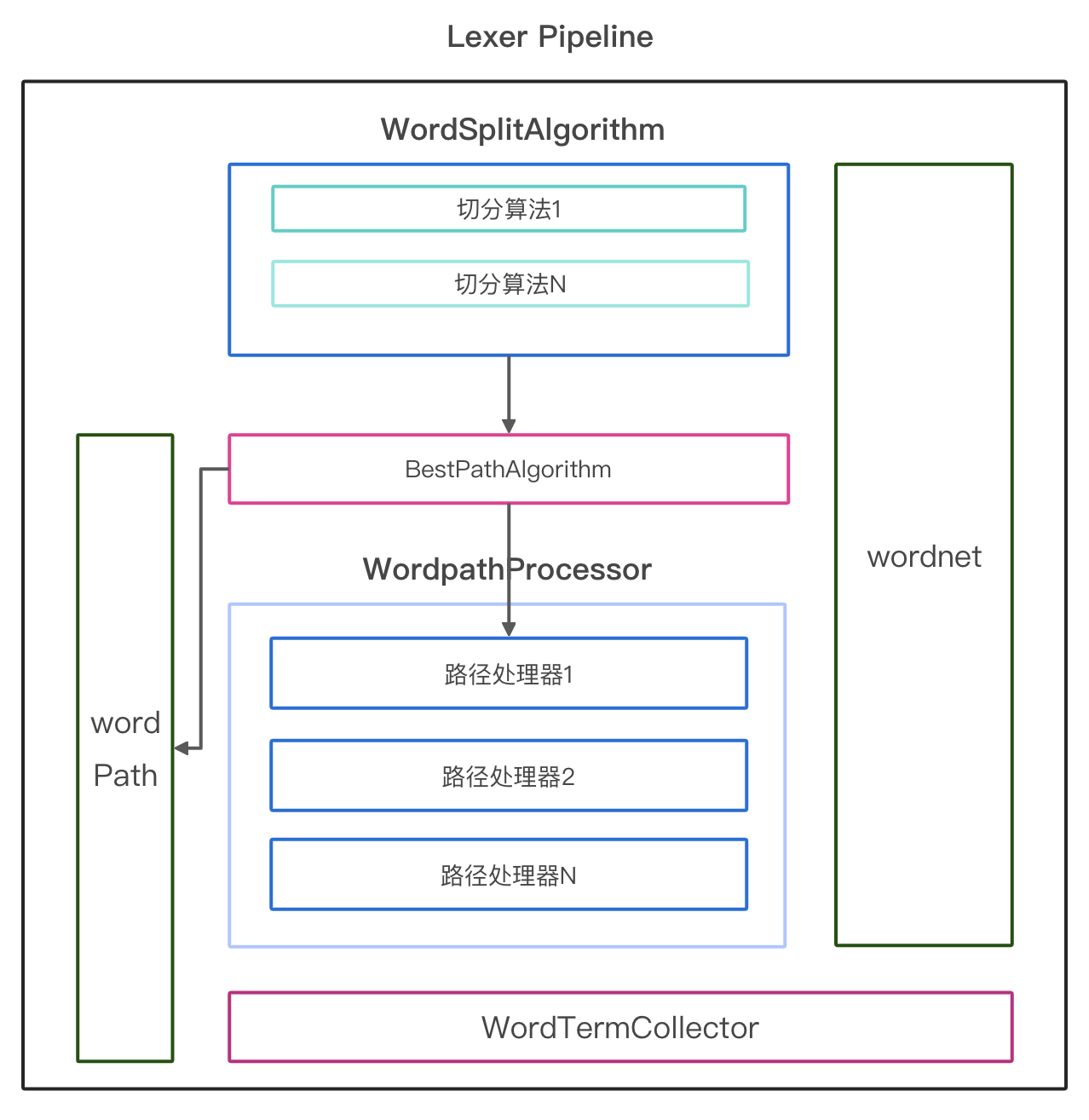
-
WordSplitAlgorithm: 基础切词算法,词典、感知机、CRF等等
-
WordpathProcessor: 对Wordpath进行调整或计算词性等操作
-
BestPathAlgorithm: 从Wordnet中选择最优路径
-
WordTermCollector: 分词收集器,可以进行索引分词、子词再切分等操作 ---
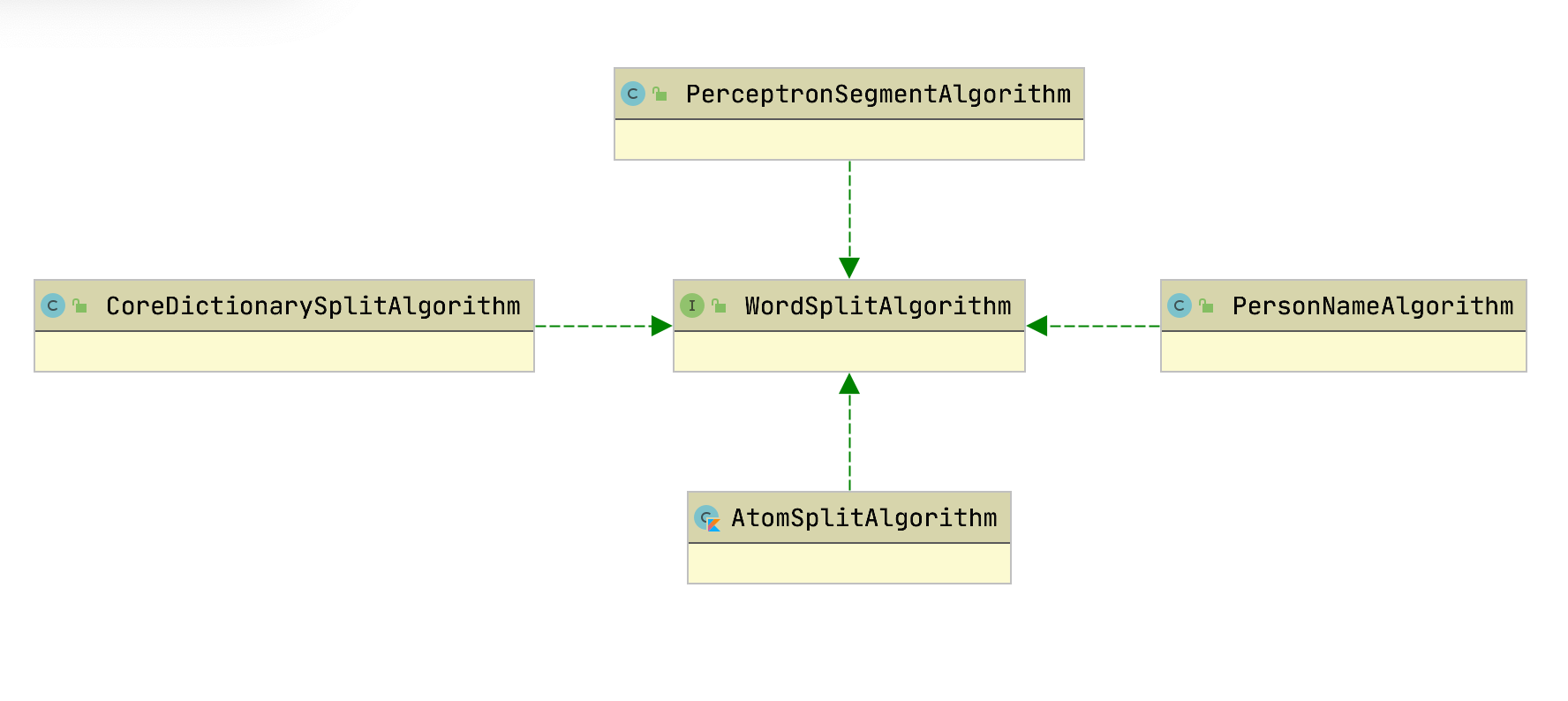
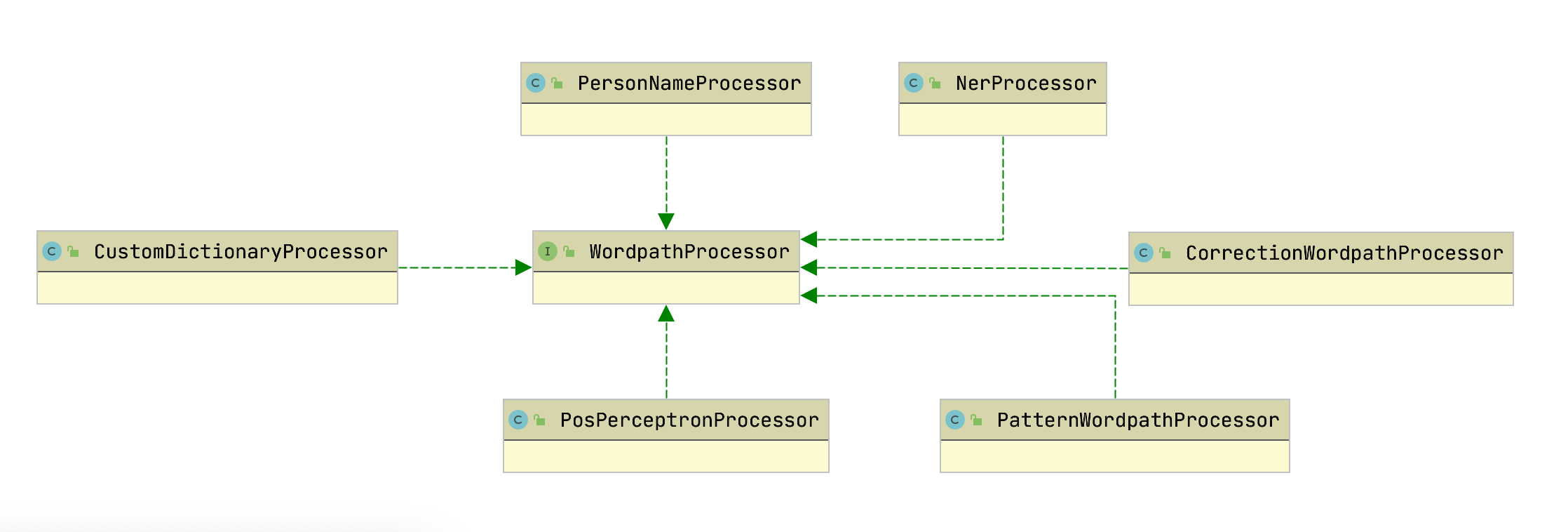
PipelineBuilder
CharNormalize
WordSplitAlgorithm
CORE
感知机
ATOM
WordpathProcessor
人名识别
NER
分词纠错
自定义词典
WordTermCollector
扩展插件
自定义分词粒度插件示例
结构化感知机框架
fastText
FastText4j是java&kotlin开发的fasttext算法库。Fasttext 是由facebookresearch开发的一个文本分类和词向量的库。
-
100%由kotlin&java实现
-
良好的API
-
兼容官方原版的预训练模型
-
支持训练&评估模型
-
支持自有模型存储格式
-
支持使用MMAP快速加载大模型
模型训练
训练分类模型
File trainFile = new File("data/agnews/ag.train");
InputArgs inputArgs = new InputArgs();
inputArgs.setLoss(LossName.softmax);
inputArgs.setLr(0.1);
inputArgs.setDim(100);
inputArgs.setEpoch(20);
FastText model = FastText.trainSupervised(trainFile, inputArgs);
-
loss 损失函数
-
hs 分层softmax.比完全softmax慢一点。 分层softmax是完全softmax损失的近似值,它允许有效地训练大量类。 还请注意,这种损失函数被认为是针对不平衡的label class,即某些label比其他label更多出现在样本。 如果您的数据集每个label的示例数量均衡,则值得尝试使用负采样损失(-loss ns -neg 100)。
-
ns NegativeSamplingLoss 负采样
-
softmax default for Supervised model
-
ova one-vs-all 可用于多分类.“OneVsAll” loss function for multi-label classification, which corresponds to the sum of binary cross-entropy computed independently for each label.
-
-
lr 学习率
-
dim 向量维度
-
epoch 迭代次数
训练数据格式:
训练数据是个纯文本文件,每一行一条数据,词之间使用空格分开,每一行必须包含至少一个label标签。默认 情况下,是一个带__label__前缀的字符串。
__label__tag1 saints rally to beat 49ers the new orleans saints survived it all hurricane ivan __label__积极 这个 商品 很 好 用 。
乘积量化压缩
分类的模型可以压缩模型体积
//load from java format
FastText qmodel = model.quantize(2, false, false);
词向量训练
支持cow和Skipgram两种模型
FastText.trainCow(file,inputArgs)
//Or
FastText.trainSkipgram(file,inputArgs)
模型评估
File trainFile = new File("data/agnews/ag.train");
InputArgs inputArgs = new InputArgs();
inputArgs.setLoss(LossName.softmax);
inputArgs.setLr(0.1);
inputArgs.setDim(100);
FastText model = FastText.trainSupervised(trainFile, inputArgs);
model.test(new File("data/agnews/ag.test"),1,0,true);
F1-Score : 0.968954 Precision : 0.960683 Recall : 0.977368 __label__2 F1-Score : 0.882043 Precision : 0.882508 Recall : 0.881579 __label__3 F1-Score : 0.890173 Precision : 0.888772 Recall : 0.891579 __label__4 F1-Score : 0.917353 Precision : 0.926463 Recall : 0.908421 __label__1 N 7600 P@1 0.915 R@1 0.915
保存模型文件
保存为自定义多文件格式:
FastText model = FastText.trainSupervised(trainFile, inputArgs);
model.saveModel(new File("path/data.model"));
为了方便生成环境发布,保存为自定义单文件格式:
model.saveModelToSingleFile(new File("path/abc.model"));
加载模型
从多文件模型文件格式加载:
//load from java format
FastText model = FastText.loadModel(new File("xxx"),false);
从单文件模型加载:
//load from java format
FastText model = FastText.loadModelFromSingleFile(file);(1)
//OR
FastText model = FastText.loadModelFromSingleFile(inputStream);(2)
1 | 指定文件路径加载模型 |
2 | 直接从InputStream加载模型,方便应用程序从classpath加载模型 |
C++原版模型加载
FastText model = FastText.loadCppModel(modelFile)(1)
//OR
FastText.loadCppModel(inputStrem)(2)
1 | 指定文件路径加载模型 |
2 | 直接从InputStream加载模型,方便应用程序从classpath加载模型 |
功能API
预测分类
List<ScoreLabelPair> result = model.predict(Arrays.asList("fastText 在 预测 标签 时 使用 了 非线性 激活 函数".split(" ")), 5,0);
词向量近邻
List<ScoreLabelPair> result = model.nearestNeighbor("中国",5);
类比
By giving three words A, B and C, return the nearest words in terms of semantic distance and their similarity list, under the condition of (A - B + C).
List<ScoreLabelPair> result = fastText.analogies("国王","皇后","男",5);
资源
-
Recent state-of-the-art English word vectors
-
Word vectors for 157 languages trained on Wikipedia and Crawl
-
Models for language identification and various supervised tasks.
综合模块
拼音
文本分类
繁简体转换
Simplified2Traditional s2t = TransformService.simplified2Traditional();
System.out.println(s2t.transform("软件和体育的艺术"));
Traditional2Simplified t2s = TransformService.traditional2Simplified();
System.out.println(t2s.transform("軟件和體育的藝術"));
摘要
文本摘要包含了两个简单TextRank的实现。
KeywordSummary keywordSummary = new KeywordSummary();
keywordSummary.keyword("text",10);
SentenceSummary sentenceSummary = new SentenceSummary();
List<String> result = sentenceSummary.summarySentences(document, 10);
相似度
还没开发。
高亮与关键字替换
对文本关键字进行高亮。
List<String> keywords = new ArrayList<>();
keywords.add("居住证");
keywords.add("居住");
Highlighter highlighter = new Highlighter(keywords);(1)
String text = "居住在上海需要办理居住证";
String text = highlighter.replace(text);
1 | Highlighter对象可重复使用 |
Highlighter内部使用了Trie结构,所以replace的时间复杂度和keywords的数量几乎无关,只对原始text扫描一次。 替换过程采用前向最大匹配算法。
另外还可以通过 QuickReplacer
类来自定义替换内容。
List<String> keywords = new ArrayList<>();
keywords.add("居住证");
keywords.add("居住");
QuickReplacer quickReplacer = new QuickReplacer(keywords);
String result = quickReplacer.replace("居住在上海需要办理居住证",
(Function<String, String>) word -> "<a href='xxx'>"+word+"</a>");
Kotlin便捷扩展函数
"居住在上海需要办理居住证".highlight(listOf("居住证","居住"))
新词发现
这个文档怎么写
高级主题
Wordnet
分词系统中需要一个数据结构来表达一段文字来多种分词可能性。距离来说"商品和服务","商品/和服/务"就是其中一个 错误的切分可能。 各种分词算法的目标就是找出最合理的切分方法。
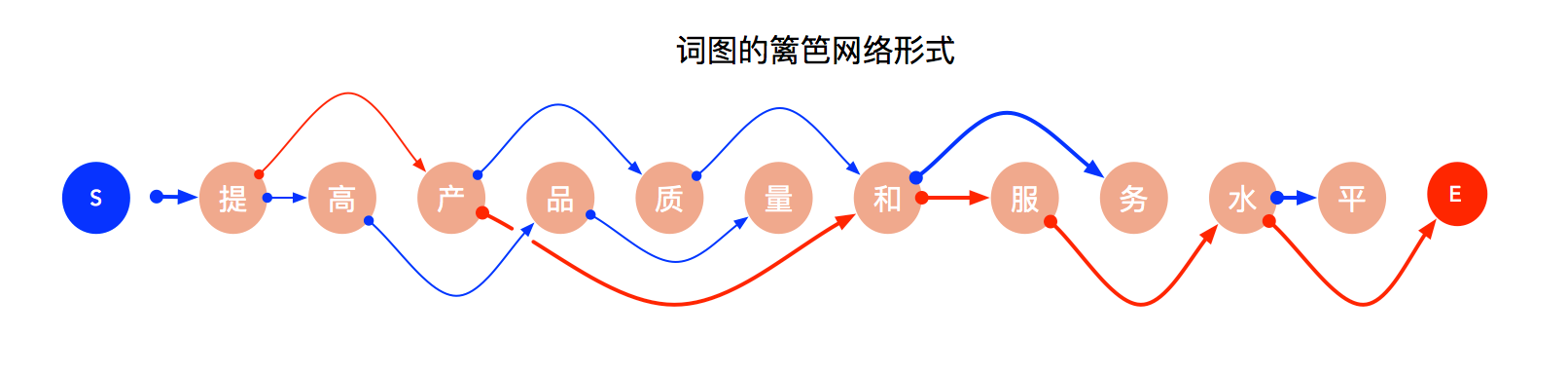
站在每个字的角度来看,会有一个或多个跳转路径。从Start节点到End节点中必定存在一个最优路径,这个路径就是 分词结果了。但是上图如果利用Node和Edge的数据结构来表达的话,性能和方便程度都很差。
Wordnet是经典的数据结构,mynlp用链表的方式实现了一个高效的Wordnet类。
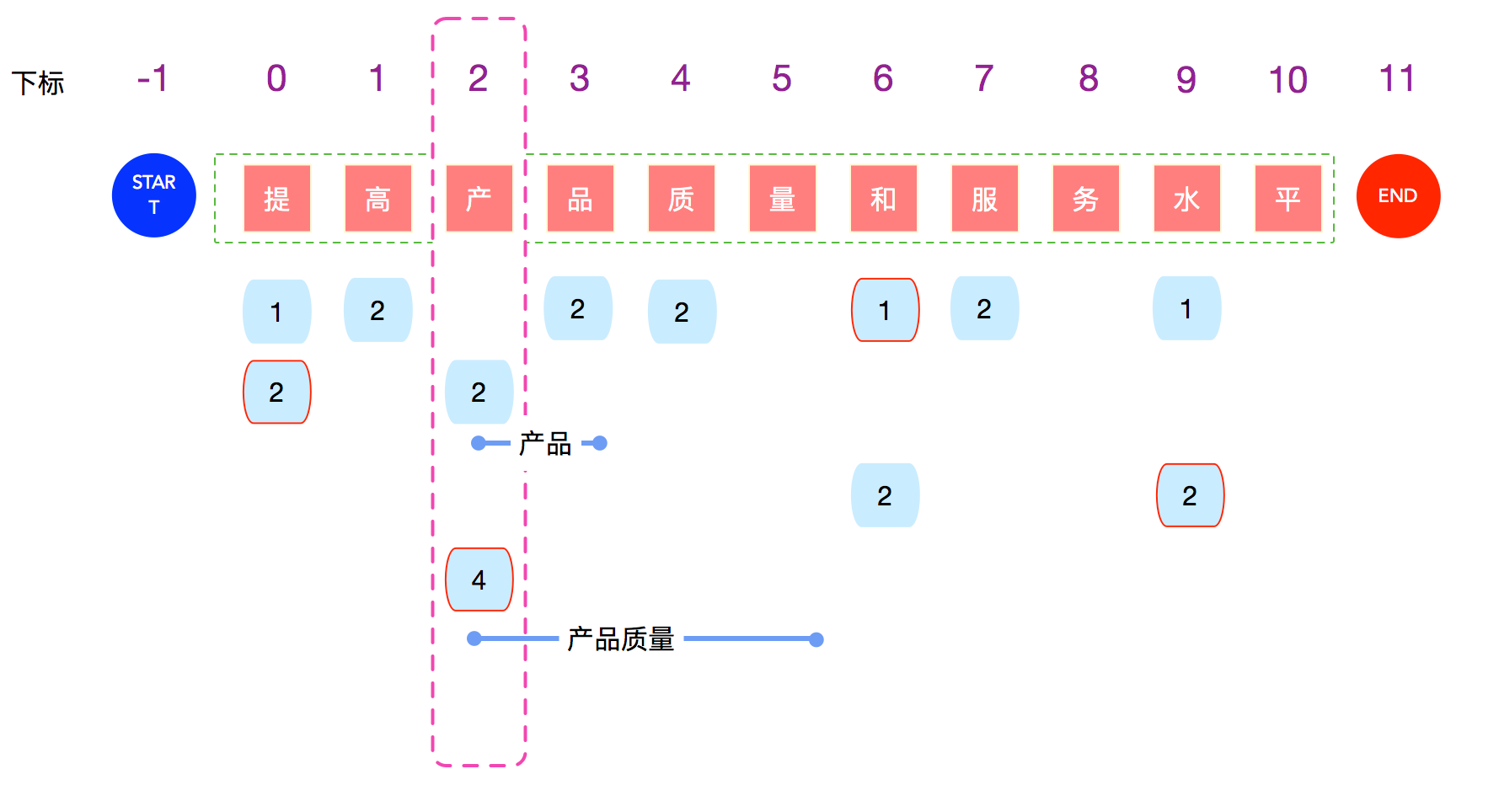
每个数字节点,表示一个边,也表示从当前这个字构成的词的长度。
对应的Java数据为:
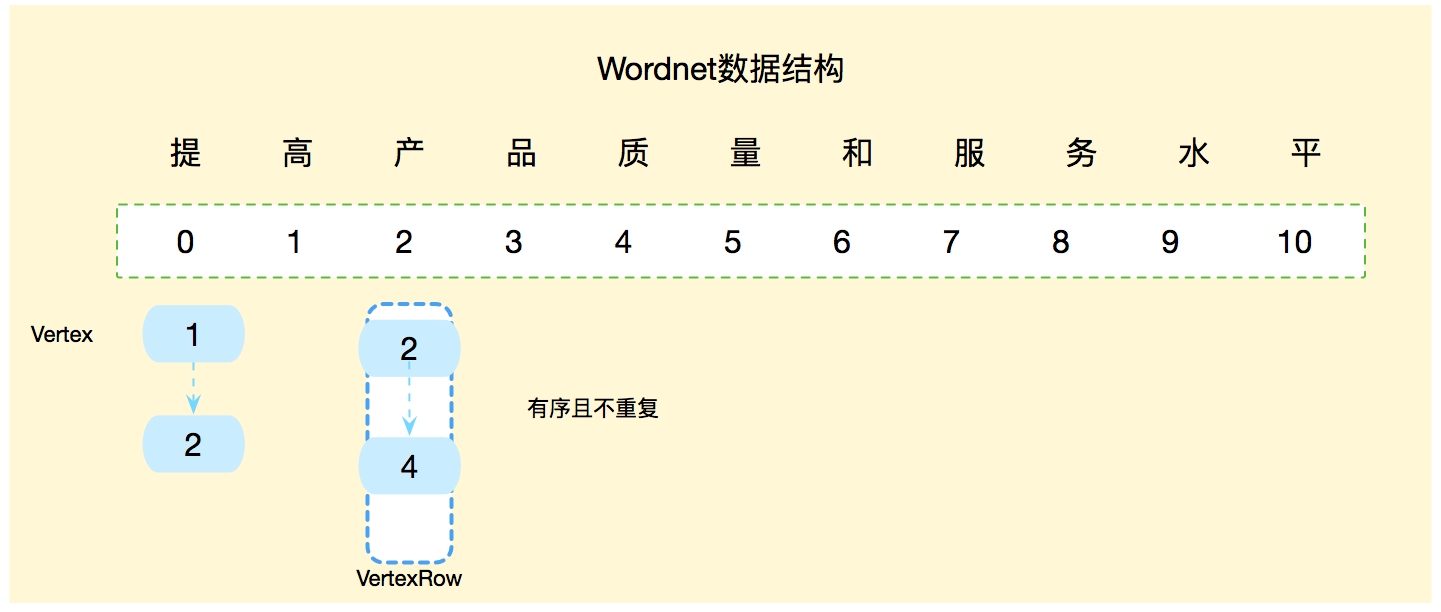
每个字对应一个`VertexRow`,每个VertexRow指向一个Vertex链表,其中Vertex链表中的数字大小一定是不可重复且有序的。
分词的基本逻辑就是填充Wordnet,使用路径选算法从多种可能性选出最佳的分词路径。
Wordpath
类Wordpath表示一个路径,如果路径不在变化,那么也就无所谓采用什么数据结构。但是在Pipeline中,不同的组件和算法还需要对这个 唯一的路径再进行修改。会涉及到很多`联合`、`打破-再联合`等操作。在List的基础上操作起来,代码非常复杂且不容易理解。
这里我们使用BitSet来表示唯一分词路径。
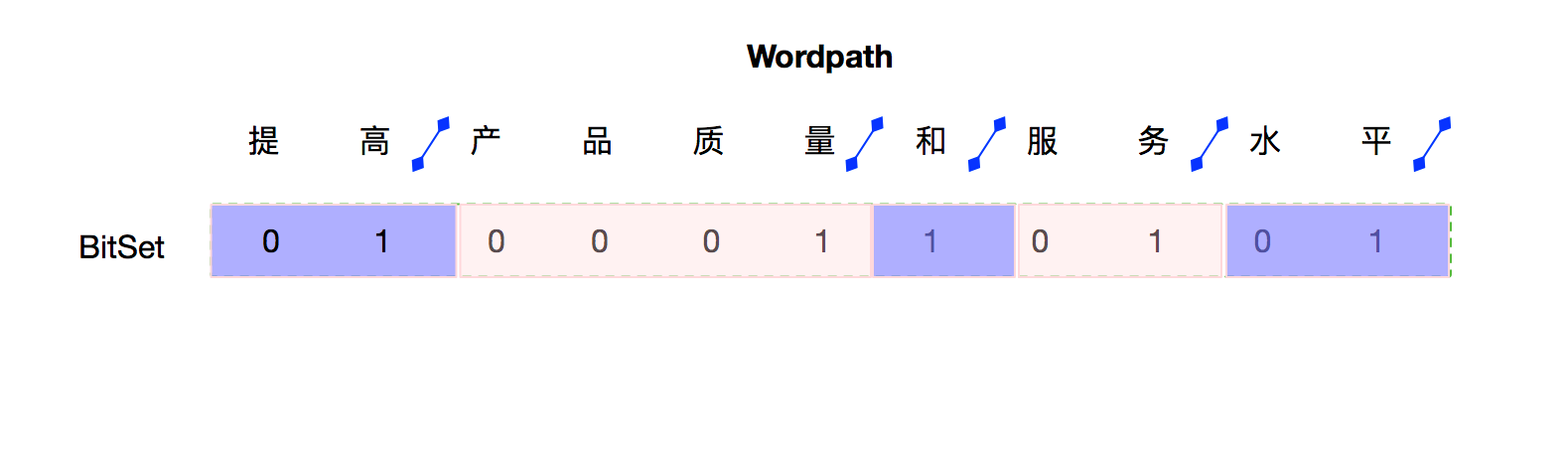
图中的字之间的斜线,表示要切断。我们用bitset中和字对应的Index,设置为true。 比如"提高"是一个词,那么设置bitset的下标1为true。
就是这么简单,使用这种数据结构的好处是,combine或者划词的操作非常简单,而且内存上消耗非常非常低。
Injector IOC容器
其他
日志
mynlp是被当做组件引入到不同的系统中,会识别当前系统使用的日志组件,进行日志输出。 这样设计的目的是兼容你项目的环境的日志配置文件 ,避免多个不同日志系统的冲突。
支持如下日志组件:
日志组件 | 说明 |
---|---|
JdkLogger |
java.util.logging.Logger |
Log4JLogger |
log4j 1.x |
Log4J2Logger |
log4j 2.x,logback |
Slf4JLogger |
slf4j |
Mynlp使用了一个Logger抽象层,系统运行时去Classpath查找各种Logger的实现类,如果找到了就会使用对应的实现。 所以你可以自由地选择Logger实现。无需配置,自动检测适配。
比如在你的工程中使用logback依赖:
compile group: 'ch.qos.logback', name: 'logback-classic', version: '1.2.3'
mynlp会自动使用logback输出日志。
资源加载
默认情况下,词典和模型文件都是通过JAR发布的,自然就是从classpath加载资源。
实际上为了支持各种使用场景,mynlp包含三个资源加载器: - File - JARFile - classpath
当你通过maven依赖导入资源文件jar后,classpath加载器会加载到这些资源。
mynlp.data
${mynlp.data}指定了文件加载根目录。
资源加载器File和JARFile是从${mynlp.data}指定的目录去加载资源文件, 默认 ${mynlp.data} = ~/mynlp.data
假设加载资源路径`core-dict/CoreDict.txt`,
mynlpEnv.loadResource("core-dict/CoreDict.txt")
-
FileLoader 尝试访问 ~/mynlp.data/core-dict/CoreDict.txt
-
JARFile 尝试访问 ~/mynlp.data/*.jar/core-dict/CoreDict.txt
设置mynlp.data目录
1.通过API设置
Mynlps.install((Consumer<MynlpBuilder>) it->
it.setDataDir("/path")
);
2.Java虚拟机系统属性
-Dmynlp.data.dir=/path
自定义NlpResourceFactory
如果你的资源存储在数据库、网络中,可以通过自定义NlpResourceFactory实现来实现。
public interface NlpResourceFactory {
/**
* 加载资源
*
* @param resourceName 格式为 dict/abc.dict
* @param charset 字符集
* @return 如果资源不存在那么返回NULL
*/
NlpResource load(String resourceName, Charset charset);
自定义的NlpResourceFactory安装:
Mynlps.install(mynlpBuilder -> {
mynlpBuilder.addResourceFactory(yourNlpResourceFactory);
});
`Mynlps.install`需要在系统启动初始化时调用。
致谢以下优秀开源项目
-
HanLP
-
ansj_seg
mynlp实现参考了他们算法实现和部分代码